Trustworthy AI: Why We Need It and How to Achieve It
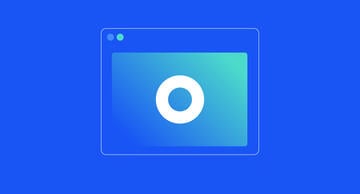
The application of machine learning to support the processing of large datasets holds promise in many industries, including financial services. In fact, over 60% of financial services companies have embedded at least one Artificial Intelligence (AI) capability, ranging from how they communicate with their customers via virtual assistants, to automating key workflows, and even managing fraud and network security.
However, AI has been shown to have a “black box” issue, resulting in a lack of understanding about how systems can work to raise concerns about opacity, unfair discrimination, ethics and threats to individual privacy and autonomy. This lack of transparency can often include hidden biases.
Here are some of the most common types of bias in AI:
- Algorithm bias: This occurs when there's a problem within the algorithm that performs the calculations that power the machine learning computations.
- Sample bias: This happens when there's a problem with the data used to train the machine learning model. In this type of bias, the data used is either not large enough or representative enough to teach the system. For example, in fraud detection it is common to receive 700,000 events per week where only one is fraudulent. Since the majority of the events are non-fraudulent, the system does not have enough examples to learn what fraud looks like, so it might assume all transactions are genuine. On the other hand, there are also cases where a vendor will share only what they consider as “risky” events or transactions (which is typically around 1% of the events). This creates the same bias problem for the training of machine learning models, since they do not have any samples of what a genuine event/transaction looks like to be able to differentiate between what is fraud and what is genuine.
- Prejudice bias: In this case, the data used to train the system reflects existing prejudices, stereotypes and/or faulty societal assumptions, thereby introducing those same real-world biases into the machine learning itself. In financial services, an example would be special claims investigations. The special investigations team often receives requests for additional investigation from managers that have reviewed claims. Because the claims are judged as suspicious by the management team, the special investigators are more likely to assume it is fraudulent than not. When these claims are entered into an AI system with the label “fraud”, the prejudice that originated with special investigators is being propagated into the AI system that learns from these data what to recognize as fraud.
- Measurement bias: This bias arises due to underlying problems with the accuracy of the data and how it was measured or assessed. Using pictures of happy workers to train a system meant to assess a workplace environment could be biased if the workers in the pictures knew they were being measured for happiness. Measurement bias can also occur at the data labeling stage due to inconsistent annotation. For example, if a team labels transactions as fraud, suspicious or genuine and someone labels a transaction as fraud but another person labels a similar one as suspicious, it will result in inconsistent labels and data.
- Exclusion bias: This happens when an important data point is left out of the data being used --something that can happen if the modelers don't recognize the data point as consequential. A relevant example here is new account fraud (i.e., cases where the account was created by a nefarious individual using a stolen or simulated identity or a new and genuine account that was taken over for the purpose of fraud). At the time of the new account opening, there are not enough data generated about the account because most modelers only start processing data after a predefined number of interactions has occurred. However, according to domain experts these first interactions that are not being immediately processed are among the most important. This simple lack of communication between domain experts and AI modelers can lead to unintentional exclusion and AI systems that are not able to detect new account fraud.
For financial institutions, the consequences of what are often unintentional biases in machine learning systems can be significant. Such biases could result in increased customer friction, lower customer service experiences, reduced sales and revenue, unfair or possibly illegal actions, and potential discrimination. Ask yourself, should organizations be able to use digital identity or social media to make judgments on your spending and likelihood to repay debts? Imagine being restricted from accessing essential goods and services just because of who you know, where you’ve been, what you’ve posted online, or even how many times you call your mother.
There are several well known cases of this, particularly in the financial domain. AI-based credit scoring is supposed to eliminate bias. But features that are included or excluded in the AI algorithms used to create a credit score can have the same effect as lending decisions made by prejudiced loan officers. As an example, the high school or university that a person attended might seem like a relevant proxy for wealth. The high school, however, may be correlated with race or ethnicity. If so, using the variable high school would punish some minority households for reasons that are unrelated to their credit risk. Studies have shown, for example, that creditworthiness can be predicted by something as simple as whether you use a Mac or a PC. But other variables, such as zip-code, can serve as a proxy for race.
Similarly, where a person shops might reveal information about their gender. Another famous example is the “Apple card” that was biased against gender. It offered significantly different interest rates and credit limits to different genders, giving large credit limits to men as compared to women. With traditional “black box” AI systems, it would be challenging to analyze and understand where this bias originated.
So, what is the solution? How can we be assured that the AI systems used are bias-free? The answer comes in the form of Trustworthy AI.
What is Trustworthy AI?
Trustworthy AI is a term used to describe AI that is lawful, ethically adherent, and technically robust. It is based on the idea that AI will reach its full potential when trust can be established in each stage of its lifecycle, from design to development, deployment and use.
There are several components needed to achieve Trustworthy AI:
- Privacy: Besides ensuring full privacy of the users as well as data privacy, there is also need for data governance access control mechanisms. These need to take into account the whole system lifecycle, from training to production which means personal data initially provided by the user, as well as the information generated about the user over the course of their interaction with the system.
- Robustness: AI systems should be resilient and secure. They must be accurate, able to handle exceptions, perform well over time and be reproducible. Another important aspect is safeguards against adversarial threats and attacks. An AI attack could target the data, the model or the underlying infrastructure. In such attacks the data as well as system behavior can be changed, leading the system to make different or erroneous decisions, and even shut down completely. For AI systems to be robust they need to be developed with a preventative approach to risks aiming to minimize and prevent harm.
- Explainability: Understanding is an important aspect in developing trust. It is important to understand how AI systems make decisions and which features were important to the decision making process for each decision. Explanations are necessary to enhance understanding and allow all involved stakeholders to make informed decisions.
AI models and their decisions are often described as “black boxes” due to the difficulty of understanding their inner workings – even by experts. The stakeholders involved in an AI system’s lifecycle should be able to understand why AI arrived at a decision and at which point it could have been different. Looking at the use case of AI-based risk assessment and fraud detection when investigating an alert for a transaction/login, there is a need to open the “black-box” and understand why an event was flagged as fraud in a way that can be interpreted by a human. Explainable AI (XAI) offers new methods to expose the important features used in the model that contribute to the high-risk score. This can help fraud analysts decide whether to take further action, and over time identify changing patterns or new types of fraud or know what to say to a user that was rejected in the process of making a payment. It is also important that the explanations should be presented in a manner appropriate for the stakeholder concerned, since different people require different levels of explanation. As Explainable AI systems are created, information officers must decide what level of stakeholder understanding is necessary. Will financial institutions need to make their AI platforms explainable to their engineers, legal team, compliance officers, or auditors? According to Gartner, by 2025, 30 percent of government and large enterprise contracts for the purchase of AI products and services will require the use of AI that is explainable and ethical. - Fairness: AI systems should be fair, unbiased, and accessible to all. Hidden biases in the AI pipeline could lead to discrimination and exclusion of underrepresented or vulnerable groups. Ensuring that AI systems are fair and include proper safeguards against bias and discrimination will lead to more equal treatment of all users and stakeholders.
- Transparency: The data, systems and business models related to AI should be transparent. Humans should be aware when they are interacting with an AI system. Also, the capabilities and limitations of an AI system should be made clear to relevant stakeholders and potential users. Ultimately transparency will contribute to having more effective traceability, auditability, and accountability.
The potential risks in AI require the involvement of critical stakeholders across government, industry and academia to ensure effective regulation and standardization. Earlier this year, the European Commission presented the ethics guidelines for Trustworthy AI. They include principles to ensure that AI systems are fair, safe, transparent, and useful for end users. Also, in the US the National Institute for Standards and Technology (NIST) is working on developing standards and tools to ensure AI is trustworthy.
In the meantime, ways to bring us closer to Trustworthy AI include:
- Conducting risk assessments: This is an emerging field with a multitude of AI applications, and the relevant risks can be challenging to identify. The European Union’s Ethics Guidelines for Trustworthy AI provide an assessment list to help companies define risks associated with AI.
- Establishing procedures: Processes for Trustworthy AI should be embedded in a company’s management processes. Existing policies should be adapted to reflect the potential impact of AI in the business and its users, especially with regard to adverse effects. The development of new compliance policies should include both technical mitigation measures and human oversight.
- Human and AI collaboration: Collaboration across disciplines, with different stakeholder groups and domain experts affected by an AI system will be important in helping define what type of system, data, and explanations are useful or necessary in a given context. Further engagement between policymakers, researchers, and those implementing AI-enabled systems will be necessary to create a trustworthy AI environment.
- Data governance: In AI systems the full pipeline development and implementation needs to be taken into account. This includes consideration of how objectives for the system are set, how the model is trained, what privacy and security safeguards are needed, what big data are used, and what the implications are for the end user and society. Explaining what training data and features have been selected for an AI system and if they are appropriate and representative of the population can contribute in counteracting common types of AI bias.
- Monitoring and controlling AI: Companies are accountable for the development, deployment and usage of AI technologies and systems. These systems need to be continuously assessed and monitored as they perform their tasks to ensure biases don't creep in over time. Using additional resources (e.g., Google's What-if Tool or IBM's AI Fairness 360 Open Source Toolkit) to examine and inspect models can help with testing, tracing and documenting their development, making them easier to validate and audit.
- Collaborations with third parties: Besides AI systems developed in house, there are cases where AI systems are procured from external business partners. In such cases there should be a commitment from all parties involved to ensure that the system is trustworthy and in compliance with current laws and regulations. Audit procedures will have to be expanded to include potential risks and adverse impacts to end users in the development of AI.
- Enhancing awareness around ethical AI: Trustworthy Artificial Intelligence is a multidisciplinary area. There is a need for increased awareness of AI ethics in the whole workforce of providers working with AI. The risks and potential impact of AI, along with ways to mitigate the risks, should be explained to all relevant stakeholders from company leaders and legal compliance officers to customer-facing employees.